In today’s rapidly evolving industrial landscape, the integration of cutting-edge technologies such as AI and Digital Twins is important to achieving superior motion control systems. These advancements enable industries to enhance automation, efficiency, and precision across various fields, such as engineering and manufacturing. Among the forefront projects driving this technological revolution is IMOCO4.E (Intelligent Motion Control under Industry 4.E), an ECSEL-funded initiative uniting 45 partners from 13 countries.
Diving into the main concept of IMOCO4.E, the project aims to create a robust knowledge base and innovative solutions for optimizing machines and production lines throughout their lifecycle. The project’s core ambition is to develop software and hardware building blocks (BBs) that are edge-to-cloud distributed, featuring standardized interfaces. These BBs form a comprehensive reference framework, incorporating the latest academic research and being designed for future enhancements.
The project revolves around two main pillars: Digital Twins and AI methodologies, including machine learning and deep learning. By employing these technologies, IMOCO4.E delivers a flexible and scalable platform, the IMOCO4.E Center, that provides a library of manufacturing solutions and applications, offering tools and solutions towards energy efficiency, configurability, traceability, and cybersecurity. The reference platform’s effectiveness was demonstrated in high-performance motion control applications across sectors such as semiconductor manufacturing, packaging, industrial robotics, and healthcare, impacting the entire value chain of the production automation and application markets.
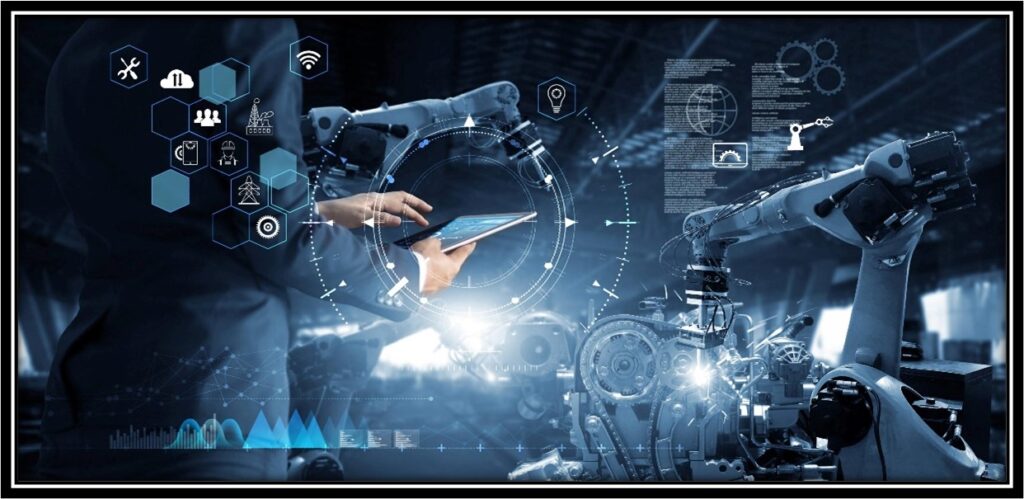
The role of predictive maintenance, data analysis and visualization in industries
Combining predictive maintenance with data analysis and visualization helps achieve proactive maintenance, optimization of performance, and extension of the lifespan of machinery, thus making industrial systems more efficient and reliable. Data analysis involves collecting and processing large volumes of operational data to identify patterns, trends, and anomalies that signal potential equipment failures. By examining this data, predictive maintenance systems can forecast when maintenance is needed, thus preventing unexpected breakdowns and optimizing machinery performance. Predictive maintenance is a proactive approach that utilizes data analysis and predictive modeling to foresee potential equipment failures before they occur. This methodology forecasts when maintenance should be performed, preventing unexpected breakdowns, reducing downtime, enhancing operational efficiency, and extending the lifespan of machinery. Visualization tools transform complex data into intuitive, real-time dashboards, enabling operators to easily interpret the data, monitor system health, and make informed decisions quickly. Visualization plays a critical role in making complex data comprehensible and actionable, highlighting key performance indicators (KPIs) and potential issues.
Digital Twins in Predictive Maintenance
Digital Twins simulate various scenarios and predict the impact of different variables on system performance. This capability is invaluable for predictive maintenance, as it allows for the identification of potential failure points and the evaluation of maintenance strategies without disrupting actual operations. A significant aspect of IMOCO4.E’s innovation lies in the integration of Digital Twins—virtual replicas of physical systems. Digital Twins offer a dynamic and interactive representation of machines and production lines, enabling real-time monitoring, simulation, and optimization. By simulating the effects of environmental conditions, and operational stress, Digital Twins models provide a comprehensive understanding of system behavior over time, facilitating proactive maintenance planning. They also enhance collaboration between design and manufacturing teams by providing a shared, real-time view of the system, fostering better communication and coordination, and serving as powerful training tools for operators.
ITML’s Dual Role in IMOCO4.E
The role of ITML in the IMOCO4.E project is comprehensive, spanning both communication and technical implementation. ITML enhances the project’s visibility through a robust communication strategy that includes developing the visual identity, designing promotional materials, executing a detailed dissemination plan, and managing publication, conference presentations, and newsletters.
On the technical front, ITML’s contributions are pivotal, particularly in data analytics, visualization, and predictive maintenance. Firstly, ITML employs Digital Twins to simulate various scenarios and predict the impact of different variables on system performance, aiding in predictive maintenance by pinpointing potential failure points without disrupting operations. This capability improves collaboration between design and manufacturing teams with real-time system views and supports virtual training for operators, boosting system reliability and efficiency. Secondly, ITML leverages advanced data analytics and visualization technologies as it uses AI-based data clustering and classification algorithms to analyze large volumes of data from motion control systems. These algorithms detect patterns and anomalies that could indicate potential failures or suboptimal performance, ensuring timely interventions through swift insights gained from edge processing. Finally, ITML deploys state-of-the-art visualization tools to create dashboards that provide operators with a clear and real-time view of system status. These dashboards enable operators to quickly understand system health and make informed decisions, significantly improving their ability to address emerging challenges.
Conclusion
IMOCO4.E represents a significant leap forward in the field of motion control, integrating AI and Digital Twins to create a flexible, scalable, and future-proof platform. ITML’s dual role in communication and technical implementation, particularly in visualization and predictive maintenance, is instrumental in realizing the project’s vision. By leveraging advanced data analytics and visualization technologies, ITML ensures that predictive maintenance is effective, enabling industries to achieve unprecedented levels of efficiency, reliability, and productivity. As IMOCO4.E continues to drive innovation, it sets the stage for a new era of industrial automation and control, benefiting a wide range of sectors and applications.
Find out more by visiting IMOCO4.E!

This project has received funding from the ECSEL Joint Undertaking (JU) under Grant Agreement No 101007311. The JU receives support from the European Union’s Horizon 2020 research and innovation programme and Netherlands, Czech Republic, Spain, Greece, Ireland, Italy, Belgium, Latvia, Portugal, Germany, Finland, Romania and Switzerland.
To find more about IMOCO4.E follow us on X and connect with us on LinkedIn.